Skin lesion recognition is primary and essential for melanoma diagnosis.
However, different melanoma appearances and low contrast between lesion region and normal region make it hard for precise segment lesion region recognition.
According to the study published in Medical Image Analysis, researchers from the Shenzhen Institutes of Advanced Technology (SIAT) of the Chinese Academy of Sciences and their collaborators from Shenzhen University proposed a novel and effective automatic segmentation method by using Generative Adversarial Networks with dual discriminators.
Researchers conducted extensive experiments to evaluate the proposed method based on the international skin imaging collaboration (ISIC) skin lesion segmentation datasets released in 2016, 2017 and 2018.
To estimate the performance of the proposed skip connection and dense convolution U-Net (UNet-SCDC) and dual discrimination mechanism, researchers carried out ablation study through 5 evaluation criterions, Accuracy, Jaccard index, Dice coefficient, Sensitivity and Specificity.
They compared the proposed dual adversarial GAN (DAGAN) with some state-of-the-art segmentation methods, including the top three methods on ISIC 2017, the top five methods on ISIC 2016 and 2018. The experimental results exhibited that DAGAN achieved the best performance on the ISIC 2017 skin lesion segmentation challenge datasets so far.
"Extensive experimental results demonstrate that our model is more generalized and achieves superior segmentation performance, which shows a bright prospect on skin lesion segmentation. We believe it can be extended to other medical image segmentation tasks in the future," said Dr. WANG Shuqiang, corresponding author of the study.
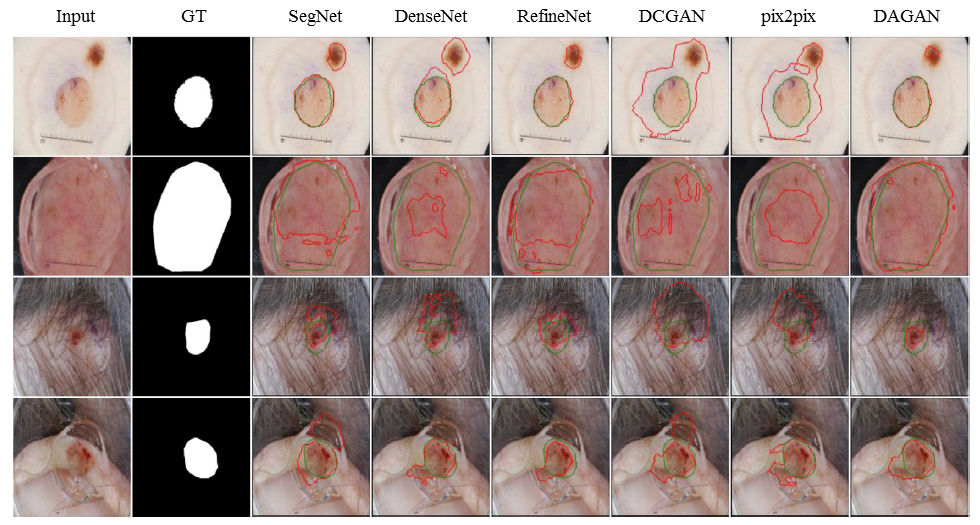
Figure. Segmentation performance comparison of different state-of-the-art networks on ISIC 2017 dataset. (Image by WANG Shuqiang)
Download the attachment:https://www.sciencedirect.com/science/article/pii/S1361841520300803