Assessment of skeletal maturity is an important tool in managing human's growth problems, especially in assisting physicians decide the best treatment for various skeletal disorders.
This task remains challenging when using machine learning method due to limited data and large anatomical variations among different subjects.
Recently, researchers from the Shenzhen Institutes of Advanced Technology (SIAT) of the Chinese Academy of Sciences and University of Hong Kong introduced an ensemble-based deep learning pipeline to automatically assess the distal radius and ulna (DRU) maturity from left-hand radiographs. The study was published in IEEE Transactions on Systems, Man, and Cybernetics: Systems . The researchers combined the dense connection mechanism with the ensemble model to improve the stability and accuracy of a skeletal maturity assessment system. The concept of densely connected mechanism was introduced in the proposed network architecture to reuse features and prevent gradient disappearance.
Results showed that the model preserved the maximum information flow and had a much faster convergence rate. By applying dense connectivity, the model could achieve better feature transfer efficiency with fewer parameters.
Besides, even trained with limited data, the proposed system had less chance to encounter the over-fitting problem. It was much easier to converge and had greater optimization efficiency.
"Our results demonstrate the superiority of the proposed model for skeletal maturity stage classification. It can be deployed in clinical environment to help orthopedists to identify the skeletal maturity via radius and ulna automatically and objectively," said Dr. WANG Shuqiang, first author of the study.
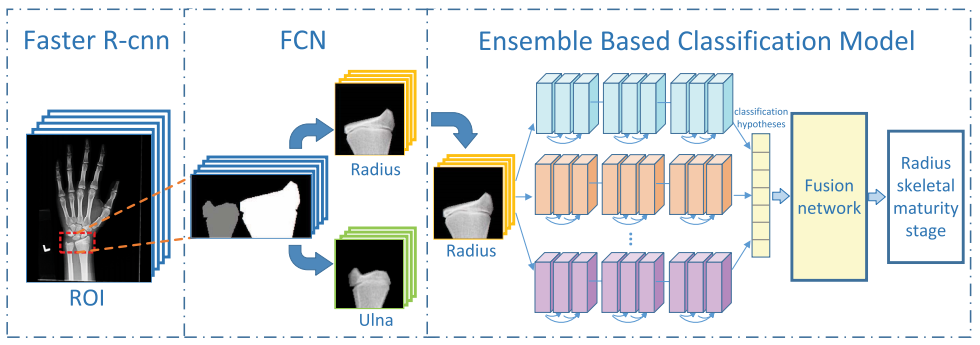
Figure. Framework of our skeletal maturity assessment system. (Image by WANG Shuqiang)
Download the attachment:https://ieeexplore.ieee.org/document/9123563